Recognizing IOA in Applied Behavior Analysis
Defining Interobserver Agreement
In the context of Applied Behavior Analysis (ABA), interobserver agreement (IOA) is defined as a measure of the extent to which two or more independent observers agree on the occurrence of a target behavior within a specified time period. If IOA is low, we cannot be certain that either observer’s record is an accurate representation of the behavior. While analyzing data collected in clinical practice , there is no requirement for researchers to establish IOA. In research studies, however, and especially in studies being used to evaluate the efficacy of interventions, it is crucial to keep in mind that bias, either conscious or subconscious, will result in an over-report of the target behavior’s occurrence.
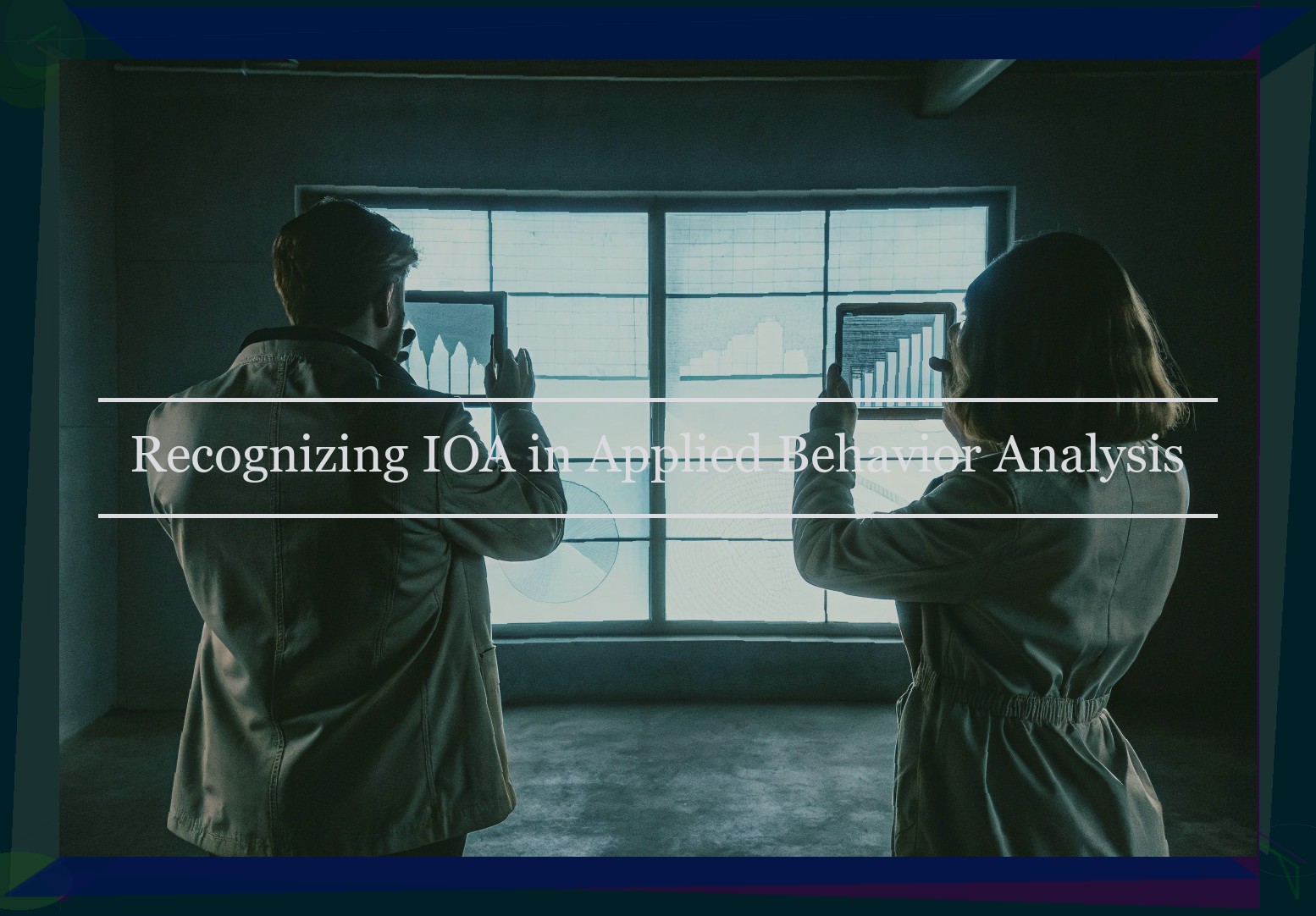
Techniques for Calculating IOA
Methods for Measurement in the field of Applied Behavior Analysis has always been an area of confusion, particularly for those new to the profession. Interobserver Agreement (IOA) is a measure of reliability or agreement between two independent raters of the occurrence and/or nonoccurrence of the target behavior that is being monitored. It is generally regarded as the primary measure of IOA. Different types of IOA include Percentage of Agreement, Cohen’s Kappa, and Intraclass Correlation.
According to McMillan & Wergin, 2013, IOA "is calculated by dividing the number of times observers agreed on a rated factor by the total number of times they both rated an individual factor". They further explain "percent agreement includes an expressed agreement of within 1 point regardless of whether the rating was above or below the median or average." The IOA index calculation is as follows: In instances where more than two raters are used the percentage of agreement is calculated by dividing the number of agreements by the total number of agreements plus disagreements.
Cohen’s kappa is another more advanced method of determining agreement. Kappa essentially adjusts for chance agreement in the data. This method is optimal when there are two raters, and has been recommended for use in IOA for behavior analysts (Fisher & Fisher, 2001).
Intraclass correlation is another alternative for analyzing agreement, particularly in instances of multiple raters. While this type of correlation is a measure of agreement between raters, it also provides a measure of reliability which will be discussed further in this section.
Troubles with Maintaining IOA
In the clinical setting, several factors can make ensuring an adequate level of agreement difficult. One such factor is the role of bias in observers’ recordings. A single observer may be influenced by their prior knowledge of or opinions about a patient. Despite this potential confounding, interobserver agreement may be preferable to relying solely on a single observer’s judgments. The other principle involved is that it is likely that reviewers will agree with themselves on subsequent recordings, so having only one observer carry out all the measurements may mean they are biased.
Unsupported biases are those that are not due to direct observation of a patient’s behavior. In this case, where there is a discrepancy between the two experts’ ensuing recordings, the result is an inflated level of agreement. This makes it possible to maintain an appearance of quality control without actually improving care. Since the goal of healthcare professionals working with patients with autism is to improve their behavioral outcomes, it is important to avoid this loophole in the observational process.
To avoid this problem, it is best practice to have observers continuously share with one another their observations of the patient. This experience is called paired ratings and is similar to the manner in which a trainer would teach their apprentice. One area in which you may not want to use this technique is in the case of students who share professors. The trainee may become so conditioned to raters in the past that they will reproduce this for the next reviewers, thus negating any effect of their personal observations. This phenomenon is referred to as the trained observer drift. Again, the best way to avoid this issue is to continue sharing observations from paired experience.
Relatedly, the complexity of the behavior being rated may have an impact on the results. That is, if the behaviors are more complex, the observers may have more difficulty applying scoring rules consistently because there is more cognitive load required. In this case, observers could draw up behavioral checklists of previously agreed upon observations for reference.
A second potential problem is a lack of experience in which raters default to a narrow set of behaviors. In other words, they may have so little background on the full range of normal behaviors a certain condition or disorder can exhibit that they try to keep their observations limited to certain specific qualities. This means they may miss subtle markers.
A third issue is related to the above problem and is a greater challenge in the assessment of complex disorders than in others, such as sleep troubles or diabetes. For example, when assessing a patient for autism, it may be very difficult to agree on the appropriate qualities to take into account in diagnosing the patient. Part of the issue is that there is no single definition of autism. As a result, it can be difficult to agree on the main qualitative dimensions. This is an ongoing area of research that will likely not be resolved in the near future.
The next problem is observer training in which there is a gap between the degree of accuracy needed for agreement and the actual level of accuracy the observers possess. Interobserver agreement is typically judged to be acceptable if the percentage of agreement is at least 80 percent. But this is not the same as saying their observations are 80 percent accurate. There is a well-known tendency of individuals to believe that they can see "the truth," and the resulting inflated self-assessment can lead to disagreement with others.
Improving IOA in Research and Application
Both researchers and clinicians can take practical steps to improve interobserver agreement beyond the training and supervision of staff. For researchers, the use of pre-study IOA calculations will help to catch problems before the project begins; a process by which researchers calculate IOA across randomly selected segments of their data before conducting the full study. Critically, this should be done by an impartial observer who is not involved in the study. In practice, this might look like the researcher providing two sessions’ worth of data to a third person (who is not involved in the study) and asking them to calculate IOA. If the IOA is low, they may consider more training or to re-examine their data collection procedures.
For clinicians, within-session IOA calculations are often the simplest way to identify issues that need to be addressed. Essentially , within-session IOA calculations involve having a second observer collect data across all target behavior during a randomly selected session. Calculating IOA for the combined session will identify segments with greater variability and will give an overall sense of the level of agreement between observers. This is a much simpler approach to collecting IOA data than collecting separate data on the same behaviors by the second observer, especially when you are dealing with clients who are not able to communicate. Additionally, it can be less time-consuming to assess ongoing agreement this way than to conduct frequent retraining or calibration sessions.
Finally, a number of electronic devices and programs exist to help clinicians collect and calculate within-session IOA and reaction time data (e.g., tray delivery, button pushing). These include video, smartphone apps, and screen capture programs. As technology continues to improve, it will play an increasingly important role in the area of IOA collection and calculation.
Examples of IOA Applications
One of our favorite examples of successful and consistent interobserver agreement occurred during a school-based functional assessment that lead to individualized intervention development for a 4th grade boy with an autism spectrum disorder. During the course of the assessment with regard to his disruptive behavior, our program lead asked the classroom teacher, "Are you ok if I sit across the desk from you at the student desk so we can have a clear view of the student in question?" The teacher immediately responded, "Sure, as long as we have our backs to each other so that neither of us can see how the other is rating the student, the results will be more trustworthy!" Think about it – two representatives of different organizations (a school district and a university) quickly agreed that if they did their assessments without knowing what the other was doing, they could better rely on the numerical calculations than if they were guessing what the other was observing. Now, when the result from the IOA calculation created a recommended individualized intervention strategy, these two organizations had a common, reliable result on which to make important decisions for a child with a disability – a rare success story. Another success story focused on a high school student’s need to reduce inappropriate classroom behavior in order to have adequate educational progress. Prior to Nickel and Dimed, the average IOA for that behavior in that classroom was 78%. During Nickel and Dimed, in addition to the standard observation form being used for instructional and behavior fidelity and school-wide reward system participation, Penelope implemented a personalized observation system that utilized IOA calculations as a part of the daily procedure for assessing the accuracy of the data. For this student, IOA for correct reporting of inappropriate classroom behavior increased from 78% to 96%. The outcome was a successfully low rate of 8% of days with inappropriate on-task behavior, contrasted with the basal rate of 20%.
Trends for the Future of Interobserver Agreement
Looking ahead, clinicians may look for trends and emerging technologies to facilitate interobserver agreement (IOA). One method of achieving this may be through highly automated and validated tools for data collection and analysis which simultaneously or automatically collect data across multiple observers and time points. For example, applications on mobile devices can be used for numerous areas of behavioral assessment and treatment such as assessment of problem behavior, or treatment delivery of a program that is contingent upon a problem behavior. These applications can be manipulated in a variety of ways to collect important variables , such as total number of occurrences for a problem behavior during a set interval, or frequency of a treatment procedure across a variable period of time. In addition, machine learning programs that are currently being developed would use a wide data set to self-optimize the collection and analysis of observer data on a large scale. These programs would utilize numerous independent user data points to determine whether observer data are significantly correlated or whether additional IOA procedures are necessary to validate the reliability of the data.